Empower your job search journey! Dive into the future with our Resume Parsing, using Machine Learning and NLP to elevate your career prospects
In the contemporary job market, the role of technology, especially machine learning (ML) and natural language processing (NLP), in streamlining recruitment processes is becoming increasingly significant.
One such area where ML and NLP are making a substantial impact is resume parsing.
This blog explores the intricate world of Resume Parsing using ML and NLP, detailing how it’s revolutionizing the way recruiters process job applications.
Table of Contents
What is Resume Parsing?
At its core, resume parsing involves converting the unstructured data of resumes into a structured format.
This process is pivotal in recruitment, enabling the extraction of key candidate information without manually sifting through each resume. Such automation leads to more efficient and energy-saving recruitment processes.
How Does Resume Parsing Work?
Resume Parser scans resumes in various formats (PDF, Word, etc.), identifies key details like name, contact information, skills, experience, and education, and organizes them into a structured database. This enables quick keyword searches and automated candidate screening.
Magical Resume Parser
Discover the powerful capabilities of the Magical Resume Parser and explore the various options available to streamline your hiring process, optimize candidate selection, and enhance recruitment efficiency.
Let’s break it down step by step.
- Step 1 Document Capture: The first step involves capturing the resume document in a digital format. Once the document is in a digital format, it can be processed by the Resume Parsing.
- Step 2: Text Extraction Once the resume document is captured, the Resume Parsing uses optical character recognition (OCR) technology to extract the text from the document.
- Step Information Extraction: After the text is extracted, the Resume Parsing employs advanced algorithms to identify and extract specific information from the document. It looks for keywords, patterns, and formats to understand the different sections and categories within the resume.
- Step 4 Data Structuring: Once the information is extracted, the Resume Parsing organizes and structures the data into a standardized format. This allows recruiters to easily compare and evaluate different resumes based on specific criteria.
- Step 5 Integration and Analysis: Finally, the parsed data is integrated into the company’s applicant tracking system (ATS) or recruitment software. This allows recruiters to efficiently manage and analyze the candidate data, making informed decisions about who to shortlist for interviews and further evaluation.
Why is Resume Parsing Important in Recruitment?
Recruiters receive hundreds of resumes for a single job posting. Resume Parsing streamlines the hiring process by reducing manual effort, improving accuracy, and ensuring fair candidate screening based on relevant qualifications rather than formatting or keyword stuffing.
Types of Resume Parsing Techniques
There are three primary resume parsing methods:
- Keyword-based Parsing: Extracts information based on specific keywords.
- Grammar-based Parsing: Uses linguistic rules to understand sentence structures.
- Statistical/NLP-based Parsing: Leverages AI to analyze context, improving accuracy.
How Resume Parsing Improves ATS Efficiency
Applicant Tracking Systems (ATS) integrate resume parsing to automate candidate filtering. This helps recruiters quickly identify the best-fit candidates, rank applicants based on relevant skills, and reduce bias in the hiring process.
Advantages of Resume Parsing
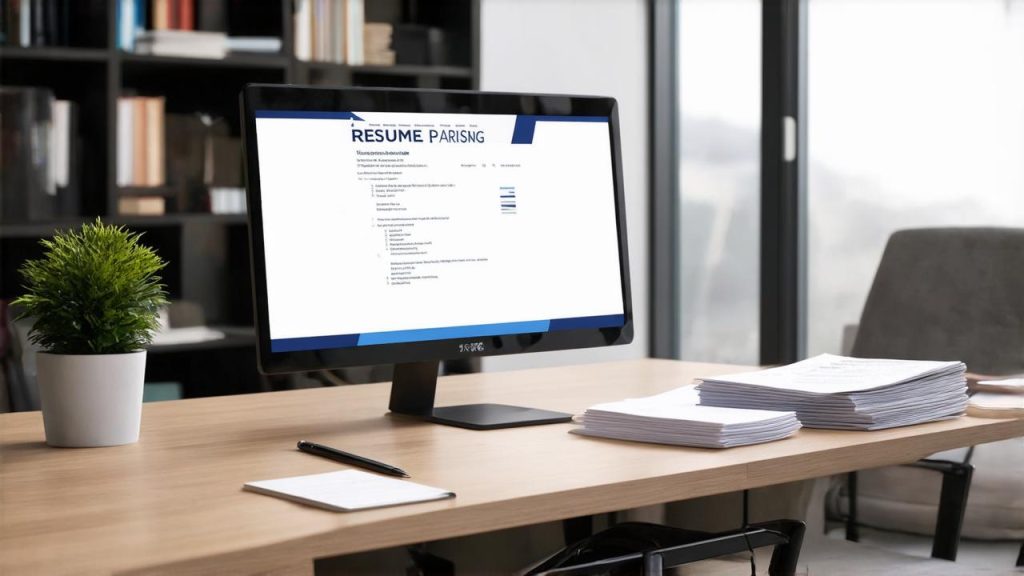
1. Efficiency in Recruitment:
- Time Savings: Automated parsing significantly reduces the time spent manually reviewing resumes, allowing recruiters to focus on more strategic aspects of the hiring process.
- Increased Productivity: Recruiters can quickly filter through a large pool of applicants, shortlisting candidates based on specific criteria.
2. Enhanced Accuracy:
- Minimized Human Error: Parsing tools eliminate the risk of manual errors in data entry, ensuring accurate and consistent information extraction.
- Objective Evaluation: By relying on algorithms, resume parsing promotes an objective evaluation of candidates, minimizing unconscious biases.
3. Improved Candidate Experience:
- Faster Response Times: Job seekers receive quicker responses, enhancing their overall experience with the hiring process.
- Personalized Communication: Recruiters can tailor their communications by leveraging parsed data, making interactions more personalized and engaging.
4. Customization for Specific Roles:
- Tailored Searches: Recruiters can easily search for candidates with specific skills or qualifications, streamlining the hiring process for specialized roles.
- Automated Screening: Parsing tools can be customized to prioritize specific keywords, ensuring that only the most relevant candidates are considered.
The Role of Machine Learning in Resume Parsing
Machine Learning (ML) has significantly transformed the landscape of numerous industries, including human resources and recruitment. Its role in enhancing the capabilities of Resume Parsing technologies cannot be overstated.
Machine learning has transformed Resume Parsing from a manual and error-prone task to an automated and accurate process.
By analyzing resumes, ML models categorize them based on various parameters like skills, experience, and educational background. This categorization helps recruiters quickly identify the most suitable candidates for specific roles.
Read More : What is a cv vs resume?
Integrating NLP in Resume Parsing
NLP’s role in resume parsing is to understand and interpret the language used in resumes. Given that natural language is often unstructured and varies across different cultural contexts, NLP techniques are essential in extracting relevant information from various formatted resumes.
How Machine Learning and NLP Enhance Resume Parsing?
Machine Learning (ML) and Natural Language Processing (NLP) are at the forefront of transforming the recruitment process through advanced Resume Parsing technology. This synergy between ML and NLP provides a dynamic, efficient, and fair approach to sorting through the vast amount of data contained in resumes. Here’s a closer look at how these technologies are enhancing resume parsing:
1.Streamlined Efficiency
ML algorithms automate the process of extracting key information from resumes, such as educational backgrounds, work experiences, skills, and certifications. This automation allows for the processing of a high volume of resumes in a fraction of the time it would take a human reviewer, significantly speeding up the recruitment process and ensuring no candidate is overlooked.
2.Improved Accuracy through NLP
NLP, a subset of ML, excels in interpreting the natural language found in resumes. It enables the Resume Parsing to understand and contextualize the unstructured text, recognizing the significance of specific terms within the industry or job role context. This results in a nuanced and accurate assessment of a candidate’s suitability for a position, enhancing the quality of candidate selection.
3. Bias Reduction
One of the significant advantages of employing ML in Resume Parsing is its contribution to a more objective and fair recruitment process. Traditional manual screening methods are prone to unconscious biases, but ML algorithms evaluate candidates based on predefined criteria related to the job requirements. This impartial evaluation promotes a fairer and more inclusive hiring process.
4. Adaptability to Changing Trends
The job market and resume formats are constantly evolving, and ML’s adaptability ensures that resume parsing technology remains effective and relevant. ML algorithms learn from new data, adapting to new patterns in resume formats and job descriptions. This ensures that the parsing capabilities continue to meet the changing demands of the job market and the ways candidates present their information.
Future of Resume Parsing: Enhancing with Advanced Models
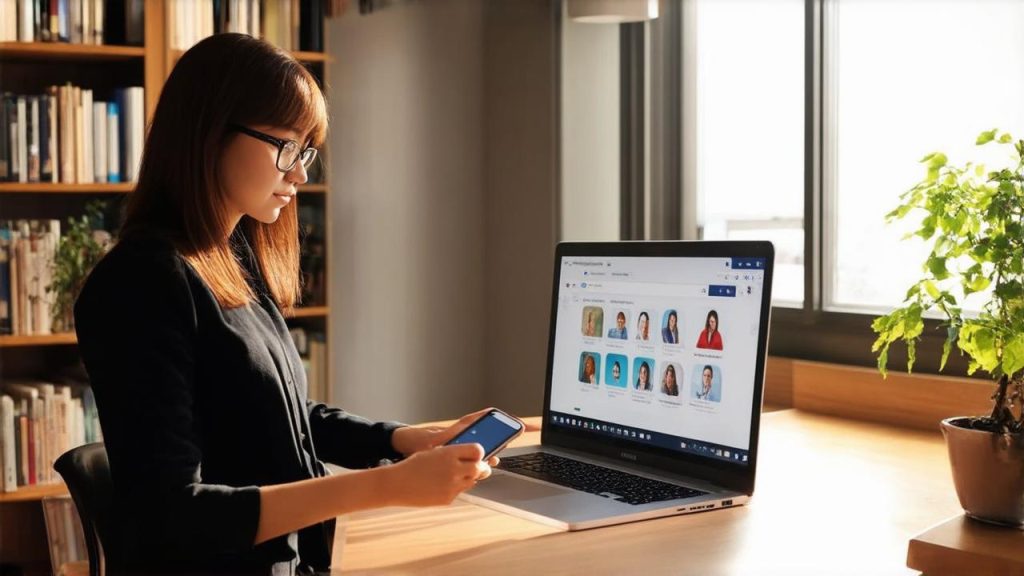
Looking ahead, the future of Resume Parsing involves enhancing the accuracy of these models, enabling multilingual parsing, and refining data extraction to include more entity types. The integration of advanced models like BERT in resume parsing is an exciting development, leveraging its pre-trained capabilities to further enhance parsing processes.
The Importance of Data for ML-Based Resume Parsing
For ML-based Resume Parsing to be effective, it requires a substantial amount of data to train the algorithms accurately. The process of creating this dataset is a blend of automated techniques and manual tagging, with tools like Doccano playing a significant role in reducing the time involved in manual tagging.
The Need for Manual Tagging
Despite the advancements in automated tagging, manual labeling remains a time-consuming yet crucial part of the data preparation process for ML-based resume parsing.
Nationality Tagging in Resume Parsing
Tagging nationality in resumes is a complex task due to the dual nature of terms like ‘Chinese‘, which can refer to both a nationality and a language. Accurate nationality tagging is crucial for parsing resumes from a global talent pool.
Leveraging BERT in Resume Parsing
The incorporation of BERT, a pre-trained NLP model, in Resume Parsing, allows for leveraging the advanced NLP capabilities of the model. This inclusion has significantly improved the efficiency and accuracy of parsing resumes, enabling the extraction of detailed information such as skills, universities attended, degrees earned, and various social media links.
Benefits of Intelligent Resume Parsing Software
Intelligent resume parsing software offers numerous benefits. It streamlines the recruitment process by automating the parsing of thousands of resumes, thereby saving time and reducing complexity. Integration with Applicant Tracking Systems (ATS) further ensures a seamless evaluation process of job applicants, aiding recruiters in making more informed decisions.
Reading More about Resume Parser vs. Manual Resume Screening
Practical Applications of Resume Parsing
In practical applications, resume parsers categorize resumes into different job categories and extract crucial information like contact details, years of experience, and relevant skills.
This automated summarization and categorization of resumes not only saves time but also enables recruiters to identify suitable candidates for specific roles quickly.
Our Advanced Resume Parser Service
In response to the growing need for efficient and accurate resume parsing, we are proud to introduce our advanced Resume Parsing service. Utilizing the latest ML and NLP technologies, our service offers unparalleled accuracy in parsing resumes, extracting essential candidate information, and categorizing it in a structured format. Our service is designed to streamline the recruitment process, enabling recruiters to focus on the most suitable candidates quickly.
Conclusion of machine learning in resume parsing
The integration of machine learning and natural language processing in resume parsing represents a significant advancement in the field of recruitment. This technology not only saves time and resources but also enhances the accuracy of candidate selection. As we continue to witness advancements in ML and NLP, the capabilities of Resume Parsing will expand further, offering more sophisticated tools for HR professionals.
FAQ About Resume Parsing
How does machine learning enhance resume parsing?
Machine learning (ML) significantly improves the accuracy and efficiency of resume parsing. By analyzing large datasets of resumes, ML models learn to identify patterns and extract relevant information such as job titles, skills, and education.
How do Resume Parsing handle different resume formats?
Resume parsers use Optical Character Recognition (OCR) to convert different formats of resumes (PDF, Word, etc.) into machine-readable text. NLP and ML algorithms then process this text to extract and structure the information.
How does a Resume Parsing integrate with Applicant Tracking Systems (ATS)?
Resume Parsing can be integrated with ATS platforms to streamline the recruitment workflow. Parsed data is organized into structured formats like JSON, XML, or CSV, which can be easily imported into ATS systems.
What is resume parsing?
Resume parsing is the process of using software to convert unstructured resume data into a structured format, such as XML or JSON. This allows the information to be easily loaded into systems like Applicant Tracking Systems (ATS) or Customer Relationship Management (CRM) platforms.